PSY 2061 Assignment Minitab and Variability
PSY 2061 Assignment Minitab and Variability
For my last several posts, I’ve been writing about the problems associated with variability. First, I showed how variability is bad for customers. Next, I showed how variability is generally harder to control than the mean. In this post, I’ll show yet one more way that variability causes problems!
Variability can dramatically reduce your statistical power during hypothesis testing. Statistical power is the probability that a test will detect a difference (or effect) that actually exists.
It’s always a good practice to understand the variability present in your subject matter and how it impacts your ability to draw conclusions. Even when you can’t reduce the variability, you can plan accordingly in order to assure that your study has adequate power.
(As a bonus for readers of this blog, this post contains the information necessary to solve the mystery that I will pose in my first post of the new year!)
HOW VARIABILITY AFFECTS STATISTICAL POWER
Higher variability reduces your ability to detect statistical significance. But how?
The probability distribution plots below illustrate how this works. These three plots represent cases where we would use 2-sample t tests to determine whether the two populations have different means. These plots represent entire populations so we know that the 3 pairs of populations are truly different. However, for statistical analysis, we almost always use samples from the population, which provides a fuzzier picture.
For random samples, increasing the sample size is like increasing the resolution of a picture of the populations. With just a few samples, the picture is so fuzzy that we’d only be able to see differences between the most distinct of populations. However, if we collect a very large sample, the picture becomes sharp enough to determine the difference between even very similar populations.
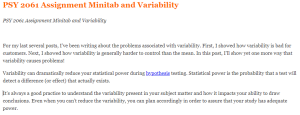
Each plot below displays two populations that we are studying. For all plots, the two populations have the same two means of 10 and 11, but different standard deviations, so the mean difference between all pairs of populations is always 1.
When the populations in these graphs are less visually distinct, we need higher resolution in our picture (i.e., a larger sample) to detect the difference.
For the high variability group, the 2 populations are virtually indistinguishable. It’s going to take a fairly high resolution to see this difference.
Click here to ORDER an A++ paper from our MASTERS and DOCTORATE WRITERS: PSY 2061 Assignment Minitab and Variability
Lopes Write Policy
For assignments that need to be submitted to Lopes Write, please be sure you have received your report and Similarity Index (SI) percentage BEFORE you do a “final submit” to me.
Once you have received your report, please review it. This report will show you grammatical, punctuation, and spelling errors that can easily be fixed. Take the extra few minutes to review instead of getting counted off for these mistakes.
Review your similarities. Did you forget to cite something? Did you not paraphrase well enough? Is your paper made up of someone else’s thoughts more than your own?
Visit the Writing Center in the Student Success Center, under the Resources tab in LoudCloud for tips on improving your paper and SI score.
Late Policy
The university’s policy on late assignments is 10% penalty PER DAY LATE. This also applies to late DQ replies.
Please communicate with me if you anticipate having to submit an assignment late. I am happy to be flexible, with advance notice. We may be able to work out an extension based on extenuating circumstances.
If you do not communicate with me before submitting an assignment late, the GCU late policy will be in effect.
I do not accept assignments that are two or more weeks late unless we have worked out an extension.
As per policy, no assignments are accepted after the last day of class. Any assignment submitted after midnight on the last day of class will not be accepted for grading.
Communication
Communication is so very important. There are multiple ways to communicate with me:
Questions to Instructor Forum: This is a great place to ask course content or assignment questions. If you have a question, there is a good chance one of your peers does as well. This is a public forum for the class.
Individual Forum: This is a private forum to ask me questions or send me messages. This will be checked at least once every 24 hours.
Important information for writing discussion questions and participation
Welcome to class
Hello class and welcome to the class and I will be your instructor for this course. This is a -week course and requires a lot of time commitment, organization, and a high level of dedication. Please use the class syllabus to guide you through all the assignments required for the course. I have also attached the classroom policies to this announcement to know your expectations for this course. Please review this document carefully and ask me any questions if you do. You could email me at any time or send me a message via the “message” icon in halo if you need to contact me. I check my email regularly, so you should get a response within 24 hours. If you have not heard from me within 24 hours and need to contact me urgently, please send a follow up text to
I strongly encourage that you do not wait until the very last minute to complete your assignments. Your assignments in weeks 4 and 5 require early planning as you would need to present a teaching plan and interview a community health provider. I advise you look at the requirements for these assignments at the beginning of the course and plan accordingly. I have posted the YouTube link that explains all the class assignments in detail. It is required that you watch this 32-minute video as the assignments from week 3 through 5 require that you follow the instructions to the letter to succeed. Failure to complete these assignments according to instructions might lead to a zero. After watching the video, please schedule a one-on-one with me to discuss your topic for your project by the second week of class. Use this link to schedule a 15-minute session. Please, call me at the time of your appointment on my number. Please note that I will NOT call you.
Please, be advised I do NOT accept any assignments by email. If you are having technical issues with uploading an assignment, contact the technical department and inform me of the issue. If you have any issues that would prevent you from getting your assignments to me by the deadline, please inform me to request a possible extension. Note that working fulltime or overtime is no excuse for late assignments. There is a 5%-point deduction for every day your assignment is late. This only applies to approved extensions. Late assignments will not be accepted.
If you think you would be needing accommodations due to any reasons, please contact the appropriate department to request accommodations.
Plagiarism is highly prohibited. Please ensure you are citing your sources correctly using APA 7th edition. All assignments including discussion posts should be formatted in APA with the appropriate spacing, font, margin, and indents. Any papers not well formatted would be returned back to you, hence, I advise you review APA formatting style. I have attached a sample paper in APA format and will also post sample discussion responses in subsequent announcements.
Your initial discussion post should be a minimum of 200 words and response posts should be a minimum of 150 words. Be advised that I grade based on quality and not necessarily the number of words you post. A minimum of TWO references should be used for your initial post. For your response post, you do not need references as personal experiences would count as response posts. If you however cite anything from the literature for your response post, it is required that you cite your reference. You should include a minimum of THREE references for papers in this course. Please note that references should be no more than 5 years old except recommended as a resource for the class. Furthermore, for each discussion board question, you need ONE initial substantive response and TWO substantive responses to either your classmates or your instructor for a total of THREE responses. There are TWO discussion questions each week, hence, you need a total minimum of SIX discussion posts for each week. I usually post a discussion question each week. You could also respond to these as it would count towards your required SIX discussion posts for the week.
I understand this is a lot of information to cover in 5 weeks, however, the Bible says in Philippians 4:13 that we can do all things through Christ that strengthens us. Even in times like this, we are encouraged by God’s word that we have that ability in us to succeed with His strength. I pray that each and every one of you receives strength for this course and life generally as we navigate through this pandemic that is shaking our world today. Relax and enjoy the course!
Hi Class,
Please read through the following information on writing a Discussion question response and participation posts.
Contact me if you have any questions.
Important information on Writing a Discussion Question
- Your response needs to be a minimum of 150 words (not including your list of references)
- There needs to be at least TWO references with ONE being a peer reviewed professional journal article.
- Include in-text citations in your response
- Do not include quotes—instead summarize and paraphrase the information
- Follow APA-7th edition
- Points will be deducted if the above is not followed
Participation –replies to your classmates or instructor
- A minimum of 6 responses per week, on at least 3 days of the week.
- Each response needs at least ONE reference with citations—best if it is a peer reviewed journal article
- Each response needs to be at least 75 words in length (does not include your list of references)
- Responses need to be substantive by bringing information to the discussion or further enhance the discussion. Responses of “I agree” or “great post” does not count for the word count.
- Follow APA 7th edition
- Points will be deducted if the above is not followed
- Remember to use and follow APA-7th edition for all weekly assignments, discussion questions, and participation points.
- Here are some helpful links
- Student paper example
- Citing Sources
- The Writing Center is a great resource
As we begin this session, I would like to take this opportunity to clarify my expectations for this course:
Please note that GCU Online weeks run from Thursday (Day 1) through Wednesday (Day 7).
Course Room Etiquette:
- It is my expectation that all learners will respect the thoughts and ideas presented in the discussions.
- All postings should be presented in a respectful, professional manner. Remember – different points of view add richness and depth to the course!
Office Hours:
- My office hours vary so feel free to shoot me an email at Dellorusso1@my.gcu.edu or my office phone is 602.639.6517 and I will get back to you within one business day or as soon as possible.
- Phone appointments can be scheduled as well. Send me an email and the best time to call you, along with your phone number to make an appointment.
- I welcome all inquiries and questions as we spend this term together. My preference is that everyone utilizes the Questions to Instructor forum. In the event your question is of a personal nature, please feel free to post in the Individual Questions for Instructor forumI will respond to all posts or emails within 24 or sooner.
Late Policy and Grading Policy
Discussion questions:
- I do not mark off for late DQ’s.
- I would rather you take the time to read the materials and respond to the DQ’s in a scholarly way, demonstrating your understanding of the materials.
- I will not accept any DQ submissions after day 7, 11:59 PM (AZ Time) of the week.
- Individual written assignments – due by 11:59 PM AZ Time Zone on the due dates indicated for each class deliverable.
Assignments:
- Assignments turned in after their specified due dates are subject to a late penalty of -10%, each day late, of the available credit. Please refer to the student academic handbook and GCU policy.
- Any activity or assignment submitted after the due date will be subject to GCU’s late policy
- Extenuating circumstances may justify exceptions, which are at my sole discretion. If an extenuating circumstance should arise, please contact me privately as soon as possible.
- No assignments can be accepted for grading after midnight on the final day of class.
- All assignments will be graded in accordance with the Assignment Grading Rubrics
Participation
- Participation in each week’s Discussion Board forum accounts for a large percentage of your final grade in this course.
- Please review the Course Syllabus for a comprehensive overview of course deliverables and the value associated with each.
- It is my expectation that each of you will substantially contribute to the course discussion forums and respond to the posts of at least three other learners.
- A substantive post should be at least 200 words. Responses such as “great posts” or “I agree” do notmeet the active engagement expectation.
- Please feel free to draw on personal examples as you develop your responses to the Discussion Questions but you do need to demonstrate your understanding of the materials.
- I do expect outside sources as well as class materials to formulate your post.
- APA format is not necessary for DQ responses, but I do expect a proper citation for references.
- Please use peer-related journals found through the GCU library and/or class materials to formulate your answers. Do not try to “Google” DQ’s as I am looking for class materials and examples from the weekly materials.
- I will not accept responses that are from Wikipedia, Business com, or other popular business websites. You will not receive credit for generic web searches – this does not demonstrate graduate-level research.
- Stay away from the use of personal pronouns when writing.As a graduate student, you are expected to write based on research and gathering of facts. Demonstrating your understanding of the materials is what you will be graded on. You will be marked down for lack of evidence to support your ideas.
Plagiarism
- Plagiarism is the act of claiming credit for another’s work, accomplishments, or ideas without appropriate acknowledgment of the source of the information by including in-text citations and references.
- This course requires the utilization of APA format for all course deliverables as noted in the course syllabus.
- Whether this happens deliberately or inadvertently, whenever plagiarism has occurred, you have committed a Code of Conduct violation.
- Please review your LopesWrite report prior to final submission.
- Every act of plagiarism, no matter the severity, must be reported to the GCU administration (this includes your DQ’s, posts to your peers, and your papers).
Plagiarism includes:
- Representing the ideas, expressions, or materials of another without due credit.
- Paraphrasing or condensing ideas from another person’s work without proper citation and referencing.
- Failing to document direct quotations without proper citation and referencing.
- Depending upon the amount, severity, and frequency of the plagiarism that is committed, students may receive in-class penalties that range from coaching (for a minor omission), -20% grade penalties for resubmission, or zero credit for a specific assignment. University-level penalties may also occur, including suspension or even expulsion from the University.
- If you are at all uncertain about what constitutes plagiarism, you should review the resources available in the Student Success Center. Also, please review the University’s policies about plagiarism which are covered in more detail in the GCU Catalog and the Student Handbook.
- We will be utilizing the GCU APA Style Guide 7th edition located in the Student Success Center > The Writing Center for all course deliverables.
LopesWrite
- All course assignments must be uploaded to the specific Module Assignment Drop Box, and also submitted to LopesWrite every week.
- Please ensure that your assignment is uploaded to both locations under the Assignments DropBox. Detailed instructions for using LopesWrite are located in the Student Success Center.
Assignment Submissions
- Please note that Microsoft Office is the software requirement at GCU.
- I can open Word files or any file that is saved with a .rtf (Rich Text Format) extension. I am unable to open .wps files.
- If you are using a “.wps” word processor, please save your files using the .rtf extension that is available from the drop-down box before uploading your files to the Assignment Drop Box.
Grade of Incomplete
- The final grade of Incomplete is granted at the discretion of the instructor; however, students must meet certain specific criteria before this grade accommodation is even possible to consider.
- The grade of Incomplete is reserved for times when students experience a serious extenuating circumstance or a crisis during the last week of class which prevents the completion of course requirements before the close of the grading period. Students also must pass the course at the time the request is made.
- Please contact me personally if you are having difficulties in meeting course requirements or class deadlines during our time together. In addition, if you are experiencing personal challenges or difficulties, it is best to contact the Academic Counselor so that you can discuss the options that might be available to you, as well as each option’s academic and financial repercussions.
Grade Disputes
- If you have any questions about a grade you have earned on an individual assignment or activity, please get in touch with mepersonally for further clarification.
- While I have made every attempt to grade you fairly, on occasion a misunderstanding may occur, so please allow me the opportunity to learn your perspective if you believe this has occurred. Together, we should be able to resolve grading issues on individual assignments.
- However, after we have discussed individual assignments’ point scores, if you still believe that the final grade you have earned at the end of the course is not commensurate with the quality of work you produced for this class, there is a formal Grade Grievance procedurewhich is outlined in the GCU Catalog and Student Handbook.